Machine learning has been a domain of the experts and we wanted infinite perspectives, infinite ways to thing, infinite ways we can outsource thinking itself. So I thought of moving this power from specialists to generalists. In the pursuit of this, my friend started making a global collaboration to start working on democratizing machine learning. It is a long term project starting June 2021 and involves researchers across Stanford, UCSD, RWTH aachen, UCSD, HEC and BITS Pilani. The genesis of the project started with the interactions we had with bell labs fellow and core member of R language and the pioneers in the field of statistics and machine learning. They also wanted to bring it to the fore, if the field is being developed at such a rapid pace we wanted everyone to contribute and move from mainframes to the era of personal computing. So, we started working on systems and a platform to make it accessible to gather data using spyders and crawlers even for IoT data in register based circuits by connecting at the exit node and finding patterns. We are contacing student clubs around the world and experts to help us make it as simple as a drag and drop interface and automate data pipelines. At american express I noticed, 80% of the time goes in correcting the models and adjusting for corrections. I wondered if we can automate this? This will help in reducing a large team size from 5 to 1 (based on the estimates we did using data models, variable models and automatically correcting for accuracy parameters for specific thresholds). Larry page had said that tough problems can be solved if we take a 20 year view rather than a 5 year view. We are workign slowly but steadily.
We hope to make access to data and analysis very easy for everyone.
The project then enables laymen to use machine learning tools to create whatever they want, that is literally create whatever kind of mind they want to create by changing neural arrangements, nural architectures, stacking up different systems viz. memory, decision making, tracking markers to know how different parts are using this data that can be figured out using tools being developed in the explainable machine learning domain. It is a moonshot, but one worth pursuing. Research scholars across UCSD, UCLA, Stanford, Max-planck institute and student clubs across all these institutes and Tsinghua university are working on the goal of democratising data science.We have bell labs fellow who support us in making the gaol a reality
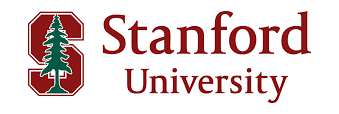
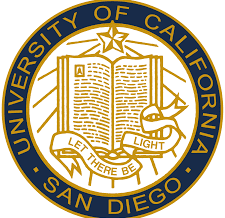
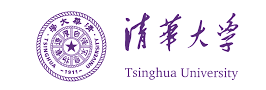
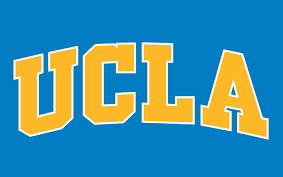
